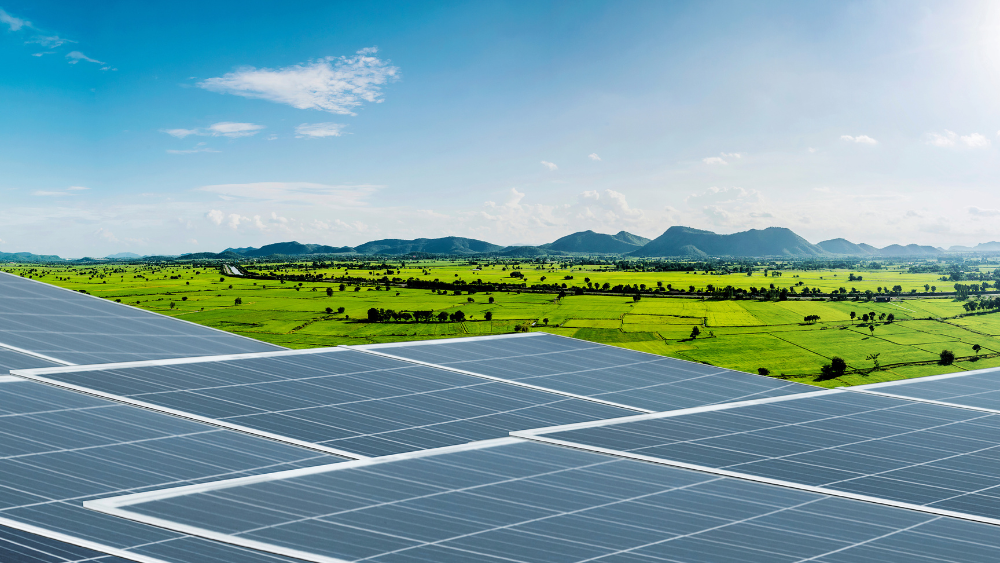
Development of Energy-efficient Perovskite Light-emitting Diodes and Solar cells
Principal Investigators & Key Members:
Le Van Quynh, PhD
This project develops next-generation LEDs and flexible solar panels for energy efficiency using emerging semiconductor materials. It focuses on creating real renewable energy devices. VinUni students gain exposure to advanced technologies in various engineering fields.